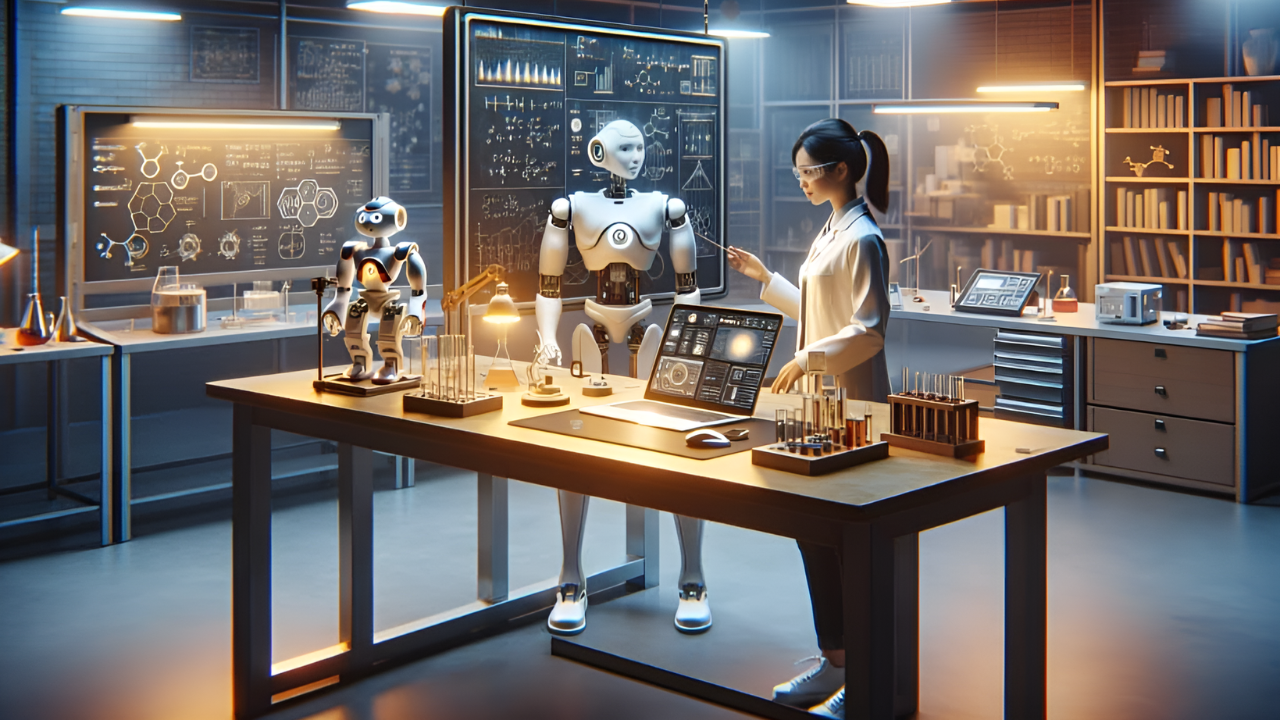
Artificial Intelligence is rapidly transforming industries, but with great power comes great responsibility. The Keeper AI Standards Test emerges as a beacon of ethical practice in this evolving landscape. It provides a structured approach to ensuring that AI systems are not only efficient but also fair and accountable. As organizations adopt advanced technologies, the necessity for rigorous testing becomes paramount. Understanding how the Keeper Standards Test works can illuminate paths toward responsible innovation and foster trust among users and stakeholders alike. Let’s dive into what makes this framework essential for ethical AI development today.
Understanding the Keeper AI Standards Framework
The Keeper AI Standards Framework is designed to address the complexities of modern AI systems. It establishes a comprehensive set of guidelines that ensure ethical practices are integrated into every phase of development. By focusing on transparency, accountability, and fairness, organizations can create AI solutions that align with societal values.
At its core, the framework emphasizes collaboration among stakeholders—developers, users, and policymakers alike. This collective approach fosters an environment where diverse perspectives contribute to building trust in AI technologies. As industries increasingly rely on artificial intelligence, adhering to these standards will be crucial for long-term success.
Core Components and Architecture
The Keeper Framework consists of three primary pillars.
- Transparency: Ensuring AI decision-making processes are transparent to stakeholders.
- Fairness: Reducing bias by identifying and mitigating it to provide equitable outcomes across diverse demographics.
- Accountability: Assigns responsibility for AI outputs while creating mechanisms to correct any errors or adverse consequences.
The Keeper Framework incorporates advanced monitoring tools, real-time auditing systems and robust data analysis pipelines to monitor and assess AI systems’ ethical compliance and ensure their responsible deployment. By taking an integrative approach towards these components, Keeper paves a path toward responsible AI deployment.
Bias Detection Methodologies
Bias in AI can have far-reaching repercussions, from reinforcing stereotypes to marginalizing marginalized groups. To detect and combat bias effectively, the Keeper Test employs advanced methodologies for detecting and mitigating it – such as:
- Data Audits: Examining training datasets to detect any imbalances or anomalies that could cause biased outputs.
- Algorithmic Scrutiny: Assessing model behavior to detect any patterns which disproportionately affect certain groups.
- Outcome Validation: Comparing AI decisions against predetermined fairness criteria to ensure fair treatment of patients.
Through various methodologies, the Keeper Test ensures that AI systems are inclusive and just, thus minimizing risks of unintended harm.
Implementing Ethical AI Testing Protocols
Implementing ethical AI testing protocols is essential for building trust in artificial intelligence. Organizations should establish clear guidelines that prioritize fairness, transparency, and accountability throughout the development process. This ensures that AI systems are not only effective but also responsible.
Key to these protocols is the identification of critical testing parameters. These can include performance metrics related to accuracy, bias detection methodologies, and user experience evaluations. By focusing on these areas, businesses can better safeguard against potential pitfalls while enhancing the reliability of their AI solutions.
Key Testing Parameters
A Keeper Test involves several key parameters that define its success:
- Accuracy: AI models should produce reliable and precise outputs.
- Transparency: Evaluates how explainable AI decisions are to stakeholders.
- Bias Mitigation: Evaluates whether the system can produce impartial outcomes.
- Adaptability: Measures an AI’s responsiveness to new data without jeopardizing ethical standards.
These parameters work together to ensure AI systems operate ethically and uphold public trust.
Fairness Assessment Metrics
Fairness metrics form the basis of ethics AI assessment. Demographic parity guarantees an equal rate of decision making in a range of different types of. The assessment framework considers various areas of study:
group fairness: The two metrics of demographic parity and equalized odds indicate whether the system maintains the same true or false positive rate for different groups of people. Research has shown that the traditional measures like equalized odds are crucial to stop the existing biases of society to persist.
individual fairness: This concept guarantees that people of similar backgrounds receive the same treatment, particularly when you use customized software. The latest research shows that the most advanced “bias-preserving” fairness techniques within computer vision systems can affect fairness through reducing the performance of the most disadvantaged group.
Error Analysis and Mitigation
Error analysis is crucial in identifying the types and sources of inaccuracies within AI systems. By systematically reviewing errors, developers can pinpoint where algorithms fail to perform as expected. This process not only helps in understanding the underlying causes but also provides insights for future improvements.
Mitigation strategies involve refining algorithms based on error patterns observed during testing. Adjustments may include retraining models with diverse datasets or implementing better data preprocessing techniques. Such proactive measures ensure that AI systems evolve, becoming more robust and reliable over time while adhering to ethical standards established by the keeper standards test.
Quality Assurance and Validation
Quality assurance and validation are crucial in the Keeper AI Standards Test. Ensuring that AI systems operate at their best requires rigorous testing against established benchmarks. This process identifies potential areas for improvement, fostering trust in AI technologies.
Reliability is another key focus during this phase. By systematically evaluating an AI system’s performance over time, developers can ensure it consistently meets user expectations. Adopting these practices not only enhances product quality but also reinforces ethical standards within the industry, promoting better outcomes for all stakeholders involved.
Performance Benchmarking
Performance benchmarking involves comparing AI systems against industry standards and predetermined benchmarks to ensure optimal functionality. Key aspects include:
- Speed and Efficiency: Assessing the time taken to process inputs and deliver outputs.
- Scalability: Assessing the system’s ability to handle large volumes of data without degradation.
- Robustness: Ensuring that an AI system performs consistently under different environmental conditions.
Benchmarking helps organizations identify areas for improvement and ensure that AI solutions meet performance expectations.
Reliability Testing
Reliability testing examines an AI system’s ability to function consistently and predictably over time. Key considerations of reliability testing include:
- Stress Testing: Simulating extreme conditions to assess system resilience.
- Error Rate Analysis: Monitoring frequency and severity of errors.
- Uptime Metrics: Measuring system availability and operational continuity.
These tests ensure that AI systems remain reliable and trustworthy when put through real-life scenarios.
Industry-Specific Applications
The Keeper AI Standards Test is crucial across various industries, ensuring that AI systems meet ethical and operational benchmarks. In healthcare, it helps maintain patient safety and compliance with regulations while promoting transparency in decision-making processes.
In financial services, the test assesses algorithms to prevent biases in lending or investment decisions. Meanwhile, manufacturing benefits from stringent quality control measures driven by AI insights. Ensuring adherence to keeper standards fosters trust and reliability across these sectors, ultimately enhancing overall performance and accountability in AI applications.
Healthcare AI ComplianceError Analysis and Mitigation
Healthcare AI compliance is crucial for maintaining patient safety and privacy. The Keeper standards test ensures that AI systems adhere to health regulations, safeguarding sensitive data while providing accurate diagnostics and treatment recommendations.
By implementing rigorous testing protocols, healthcare organizations can identify potential biases and inaccuracies in AI algorithms. This fosters trust among patients and practitioners alike, ultimately enhancing the effectiveness of AI solutions in improving health outcomes.
Financial Services Implementation
AI systems in financial services play a pivotal role in decision-making processes such as loan approvals and fraud detection. The Keeper Test helps ensure this.
- Transparency: Decisions should be explained clearly to regulators and customers.
- Fairness: Algorithms do not discriminate based on race, gender, socioeconomic status or any other factor.
- Accuracy: Predictions should be accurate and minimize false positives or negatives.
Financial institutions that employ this strategy are better able to build customer trust while complying with industry regulations.
Manufacturing Quality Control
AI has transformed manufacturing’s quality control procedures totally. Audi’s Neckarsulm facility uses machine vision to perform spot welding inspection. The technology can have cut the cost of labor by 30% to 50 percent when compared with traditional methods of inspection. This technology allows:
- Live analysis of the production process
- Automated defect detection
- Capabilities for predictive maintenance
- Quality analysis based on root causes concerns
AI used in quality control of manufacturing is astonishing in its efficacy and accuracy. The systems can detect tiny imperfections more accurately than human inspectors. The result is lower waste, higher product quality and more efficient production processes.
Conclusion
The Keeper AI Standards Test is a vital tool for ensuring ethical and reliable AI practices. By focusing on fairness, transparency, and accountability, it helps organizations build trustworthy and responsible AI systems. Its applications across industries like healthcare, finance, and manufacturing highlight its importance in promoting innovation while safeguarding societal values. The Keeper Test sets a standard for ethical AI, fostering trust and paving the way for a future where AI benefits everyone responsibly.